ML4Sci #13: An Interpretable Mortality Prediction Model for COVID-19; ML-revealed Statistics of Li-battery Cathode Failure; Accelerated Discovery of CO2 Electrocatalysts using Active ML
3 ML4Sci papers from the Nature family of journals published in May 2020
Hi, I’m Charles Yang and I’m sharing (roughly) weekly issues about applications of artificial intelligence and machine learning to problems of interest for scientists and engineers.
If you enjoy reading ML4Sci, send us a ❤️. Or forward it to someone who you think might enjoy it!
As COVID-19 continues to spread, let’s all do our part to help protect those who are most vulnerable to this epidemic. Wash your hands frequently (maybe after reading this?), check in on someone (potentially virtually), and continue to practice social distancing.
An Interpretable Mortality Prediction Model for COVID-19
Published May 14, 2020
This paper comes from University in Wuhan, and was published in Nature Machine Intelligence, with a 2 month received-to-published turnaround (which is incredibly fast, for a Nature journal).
Using time-series data of blood samples from 485 COVID-19 patients, the authors developed an interpretable decision tree for mortality prognostication, in order to determine which patients are in need of critical care. An xgboost model was used, which is an tree-boosting ensemble model that has exploded in popularity recently, especially on data science competition platforms like Kaggle. First, xgboost is trained on 75 different biomarkers to accurately forecast mortality. Then, feature importance is used to determine the top 3 features and a decision tree is trained based on these 3 features. The final decision tree model is shown below and achieved greater than 95% AUC score on the cross-validation set.
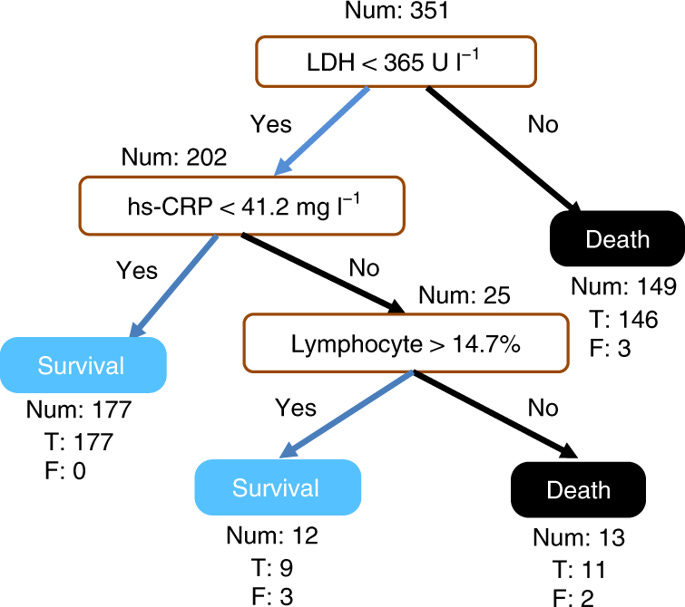
Takeaways:
Interpretable models are important and useful. Don’t look down on tree’s!
Machine learning models can help test scientific hypotheses. If a model can fit on these 3 features without loss in accuracy, then maybe it might suggests something about what is necessary and sufficient to describe some physical phenomena.
From the acknowledgements section: “We would like to dedicate this paper to those who have devoted their lives to the battle with coronavirus.”
ML-revealed Statistics of Li-battery cathode failure
Published May 08, 2020
Composite cathodes for Li batteries are usually composed of nanoparticles in a carbon binder/matrix. As a battery is cycled over time, the particles in the cathode degrade, which limits the total charge storage capacity and overall battery lifetime. Understanding this degradation process and how it affects battery performance is therefore critical for designing better batteries.
Part of this process involves particle detachment and fracturing, which affects electrical conductivity. With hard X-ray nanotomography, the researchers are able to construct 3D maps of the cathode over time. However, these tomographic maps of the cathode are large and contain particles in a variety of conditions. Traditional image segmentation models are unable to automatically label the particle degradation condition. Given that there are over 650 particles in their dataset, manually performing this would be expensive. Instead, the researchers use Mask R-CNN, a popular image segmentation model, and fine-tune it on their dataset of labelled particles. The CNN is able to improve on traditional image segmentation models, as shown below.
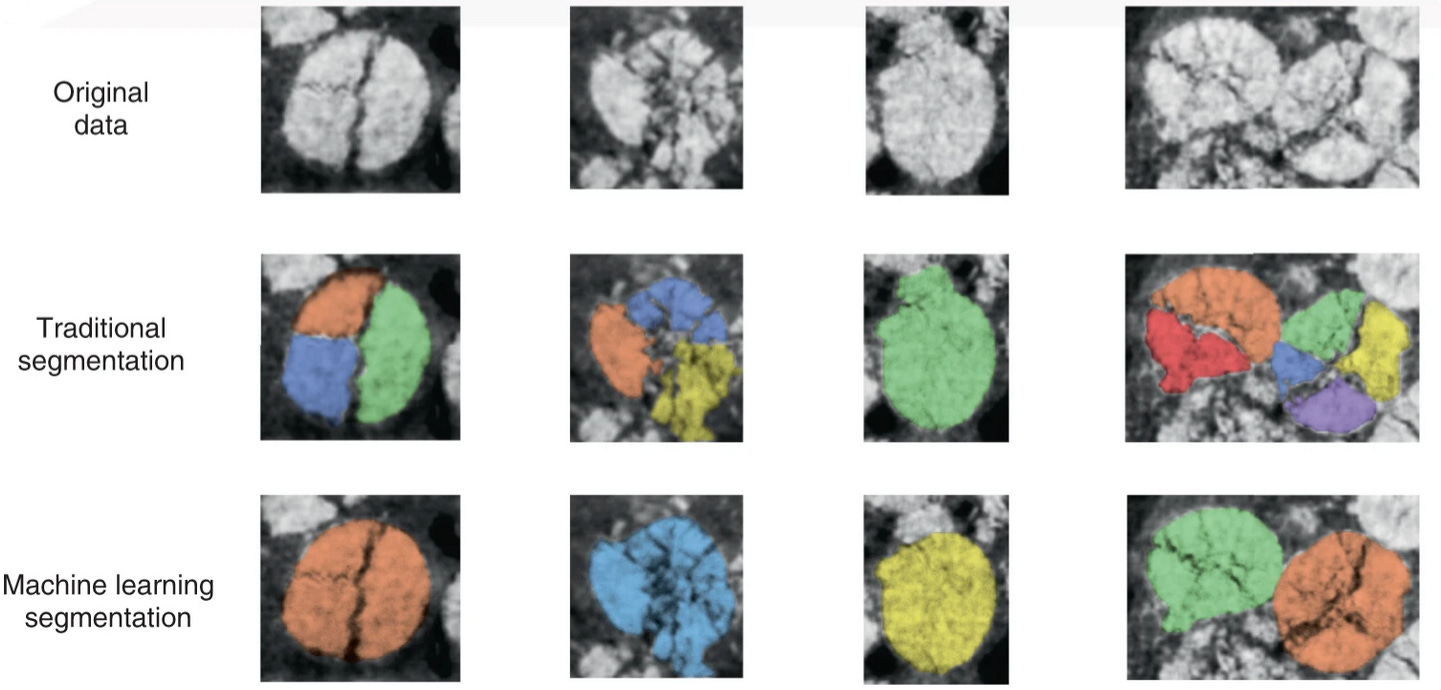
By automatically labelling the composite particles in the cathode, researchers are able to efficiently analyze distributions of particle sizes and detachment degree with respect to battery operating conditions. For instance, they conclude that fast-cycled particles have higher degrees of detachment from the binder and that smaller particles have broader distributions of detachment degree.
Takeaways:
Advances in spectroscopy, numerical simulations, or manufacturing usually open up much larger exploration spaces. Machine learning can be one way to efficiently analyze and explore these spaces.
Machine learning can be used to accelerate different components of scientific inquiry. Applications range from the coarse-scaled “inverse-design” or “fit-and-predict” to the more fine-grained, like this work, that use ML in only a small component of a larger investigation.
🔒Accelerated Discovery of CO2 Electrocatalysts using Active Machine Learning
Published on May 13, 2020
This paper is a tour de force from Ed Sargent’s group at UToronto, which is known for building state of the art photovoltaic systems and catalysts, in collaboration with researchers at Carnegie Mellon, the EECS department at UToronto, and Hsinchu, Taiwan.
Motivation and Background
CO2 electrocatalysis uses electricity to catalytic convert CO2 into commercially valuable products. The lowest hanging fruit, chemically speaking, is to convert CO2 into CO, which, when added with H2, can form methane. However, the most valuable commercial product that can be directly obtained from electrocatalysis of CO2 is ethlyene, C2H4, which is a base ingredient in a variety of chemical processes. However, it is also difficult to obtain catalytically, requiring a number of intermediate chemical reactions. Currently, copper (Cu) is understood to be the best element for CO2 to ethylene conversion, but a wide chemical space of copper-metallic systems remains to be explored. This work uses machine learning to efficiently explore that space computationally, followed by local experimental exploitation of the proposed system.
Active Machine Learning
To discover new electrocatalysts, this paper begins by scraping copper-based metallics from the Materials Project. This is not the first work we’ve covered that builds off of the Materials Project API; another piece of evidence that open-source software helps enable innovation in ML4Sci.
Starting from 244 different copper-based metallics, they generate 12,229 different crystal surfaces, resulting in 228,969 different adsorption sites. By sampling Density Functional Theory (DFT) calculations to train a machine learning model, combining prior knowledge of what criteria are needed for good electrocatalysts, and using active machine learning (they refer to it in the paper as “machine learning prioritization”), only 4000 DFT calculations in total are performed in order to converge to the optimal Cu-metallic system, which is proposed to be Cu-Al. t-SNE is used to visualize the best Cu-metallic compounds and understand why Cu-Al is the best (Al binds to CO too weakly, Cu binds to CO too strongly, Cu-Al acts as a good middle bridge).
Experimental Exploration
Using pure Cu as the benchmark, they use a variety of experimental techniques (thermal evaporation, co-sputtering+etching) to synthesize the Cu-Al catalyst and demonstrate that it indeed has better conversion efficiency of CO2 to ethylene, while minimizing unwanted byproducts. The authors also combine more advanced DFT and experimental spectroscopy to confirm the origins and mechanisms of Cu-Al’s superior ability to catalytically convert CO2 to C2H4.
Finally, the Sargent group builds off of their 2018 Science paper that showcased a novel electrocatalyst system for ethylene production and demonstrate that even with this advanced platform, Cu-Al outperforms Cu at ethylene selectivity.
Takeaways:
This paper is a great example of global ML-guided computational exploration, followed by local experimental optimization. Who knows what new material systems we will discover through AI?
In order to accelerate material discovery and design, we need agreed upon, open-source software platforms and numerical implementations of models. This is not just true of material science, but of optics, chemistry, particle physics, etc.
While machine learning is able to explore design space efficiently, experimental synthesis exploration is still manual and hand-tuned. In addition, we are often unable to enforce synthesizability constraints, either in terms of manufacturing precision or thermodynamics.
[paper]
📰In the News
What does OpenAI plan to do with the supercomputer? Probably build even bigger natural language models: OpenAI releases new GPT-3, which weighs in at a massive 175B parameters
“AI Software Gets Mixed Reviews for Tackling Coronavirus”[🔒WSJ]
Modelling COVID-19: commentary from Nature Physics
U.S. lawmakers unveil a bold $100 billion plan to remake NSF
Google AI Blog: Federated Analytics for Data Science
Insitro raises $143M for intersection of Biology and AI
Amazon in talks to buy Zoox, self-driving car startup[WSJ]. The financial crunch caused by COVID-19 is leading to lots of startups going under and big companies getting bigger.
10 tips for research and a Ph.D from NLP guru, Sebastian Ruder. At the end, it includes a “References and Inspiration” section, that points to many well-known blog posts on “How to do a ML PhD”
Thanks for Reading!
I hope you’re as excited as I am about the future of machine learning for solving exciting problems in science. You can find the archive of all past issues here and click here to subscribe to the newsletter.
Have any questions, feedback, or suggestions for articles? Contact me at ml4science@gmail.com or on Twitter @charlesxjyang