ML4Sci #25: A special issue on Batteries x AI
Charging up AI for Batteries - a crossover post from Intercalation Station
Hi, I’m Charles Yang and I’m sharing (roughly) weekly issues about applications of artificial intelligence and machine learning to problems of interest for scientists and engineers.
If you enjoy reading ML4Sci, send us a ❤️. Or forward it to someone who you think might enjoy it!
As COVID-19 continues to spread, let’s all do our part to help protect those who are most vulnerable to this epidemic. Wash your hands frequently (maybe after reading this?), wear a mask, check in on someone (potentially virtually), and continue to practice social distancing.
I’m excited to be sharing an essay I wrote in collaboration with Andrew and Nick over at Intercalation Station, a monthly newsletter covering the latest industry and research news for batteries. Check it out if you want to keep up with the world of energy storage!
🔋Charging up AI for Batteries
AI is reshaping our society and that is beginning to include the way we do science. But the problems that concern scientists and engineers are markedly different from those that occupy Silicon Valley. The unique intersection of AI technology and scientific problems is endowed with novel problem spaces and diverse opportunities. This forms one of the core hypotheses of my newsletter ML4Sci, where I write about breakthrough research in machine learning for the sciences.
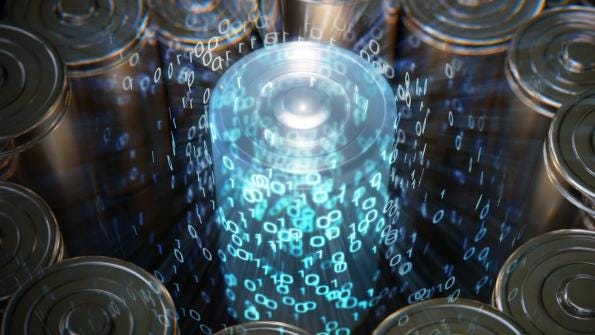
In this newsletter issue, I analyze how AI will disrupt the battery space. Specifically, I’ll talk about how AI is changing the way we discover, innovate, and develop batteries, and how it is changing the way we use them.
Battery Discovery
One mitigating factor to widespread battery adoption is the slow pace of battery R&D, from discovery to commercialization. AI promises to be one method of accelerating the discovery process for new battery materials.
We’re already beginning to see commercial success and interest in this area. For instance, battery startup Cuberg used a ML platform developed by Aionics to speed up the electrolyte development process. Kobold Metal is another startup that is using AI to sift through massive amounts of geological data to identify untapped Cobalt deposits.
In academia, there has been an explosion of materials data in the past decade, as well as unified platforms for hosting such data. The Materials Project is perhaps the best example of a standardized repository of simulated inorganic crystalline materials, along with their own Battery Explorer app. High-throughput simulations, guided by AI, can serve as a first-pass test for possible next-generation materials for batteries. For instance, Argonne National Lab used AI to accelerate electrolyte candidate screening; another group at Stanford used ML to identify Li-conductors from the Materials Project and benchmark the model’s performance with graduate students.
In addition to being able to efficiently sift through massive amounts of simulated data, AI is also able to extract relevant information from text. A paper published in Nature last year from Berkeley showed that a simple Doc2Vec algorithm was able to predict material properties of different compounds before they were experimentally validated. Massive literature scraping is also providing a way for AI to propose synthesis routes for theoretically predicted materials.
More broadly, AI is accelerating the way we do computational simulations, high-throughput experiments, and characterization. By studying how AI is affecting the way we do science as a whole, we can better predict the ways AI will affect batteries in particular. For instance, this paper uses machine learning to label the huge amount of data collected by X-ray tomography, enabling the authors to extract greater insight into the Li-ion cathode degradation process. But this isn’t really a Battery x AI use case - it’s a more broad example of how AI can help us better understand high-resolution spectroscopic data. On the other hand, robotics/automation + artificial intelligence is already being used in other fields, particularly for 3D printing composites and photocatalysts. By studying trends in other fields, we might be able to predict further in advance all the ways AI will continue to drive progress in battery development.
Battery Use
A fully commercialized battery is naked without a strong integration into a user system, be it an electric vehicle or a utility-scale renewable energy project. How to control complex electrochemical systems at a variety of scales, from the pouches in a smartphone to entire battery grid farms, is a difficult problem.
Already, recent research has demonstrated a broad umbrella of applications for AI in battery use, from identifying fast charging protocols (six-step, ten-minute), state-of-charge estimation, and predicting battery cycle life before capacity degradation is reached. Indeed, while there is a huge space of battery chemistries to explore, it could be argued that there is equal performance margin left to squeeze out of current Li-ion batteries simply by optimizing the way we use them.
An increasingly dynamic power grid will make battery control optimization increasingly complex and multidimensional - from demand response to battery health to grid stability. AI is increasingly helping us adapt batteries for a variety of scenarios and stakeholders. Commercially, companies like Tesla are already providing a suite of energy software to intelligently manage fleets of energy resources. Startups like Stem and AMS are also focusing on different verticals of the power market, using optimization systems.
Final Thoughts
AI holds great promise in helping address climate change (though it is no panacea). An increasingly interconnected and complex world requires algorithms that are able to parse the flood of data and provide actionable insights. The massive literature corpus on batteries, the vast experimental and materials space for batteries, as well as their incorporation into an increasingly dynamic power grid, are all attributes of battery research that are amenable to AI. Keep an eye out in this space for more disruption and innovation - I’m personally always excited to see how AI is changing the way we do science and discover new things.
Thanks for making it to the end and welcome to all our new subscribers! I’m excited to be working on several different initiatives to help connect the ML4Sci community (so that we can talk to each other besides just through onerous peer review processes). I’m also hoping to provide an issue about some of the explicit hypotheses and themes that we’ve seen throughout this newsletter, so stay tuned!
P.S. if you enjoyed this essay, why not share it?
Thanks for Reading!
I hope you’re as excited as I am about the future of machine learning for solving exciting problems in science. You can find the archive of all past issues here and click here to subscribe to the newsletter.
Have any questions, feedback, or suggestions for articles? Contact me at ml4science@gmail.com or on Twitter @charlesxjyang