ML4Sci #33: AI models that cost $1B USD to train; Transformers continue eating the world; Blockchain for research papers
Also, tales of ML4Sci from the Twitter-verse
Hi, I’m Charles Yang and I’m sharing (roughly) weekly issues about applications of artificial intelligence and machine learning to problems of interest for scientists and engineers.
If you enjoy reading ML4Sci, send us a ❤️. Or forward it to someone who you think might enjoy it!
As COVID-19 continues to spread, let’s all do our part to help protect those who are most vulnerable to this epidemic. Wash your hands frequently (maybe after reading this?), wear a mask, check in on someone (potentially virtually), and continue to practice social distancing.
Department of Machine Learning
Paperswithoutcode: a new platform to identify unreproducible papers
🤯NVIDIA VP predicts the next big language model is going to cost $1B USD to train. Training AI models is approaching the same order of magnitude of cost as the Manhattan project. Lots of lessons here: the democratizing access of computers and software, the monolithic scale of tech companies and the impossibly high barriers to building performant AI models, and of course, the coming shifts in the geopolitical landscape.
[Medium] ML-in-production: “How We Scaled Bert To Serve 1+ Billion Daily Requests on CPUs”@Roblox
[Arxiv] From the Google datacenters: neural architecture search optimized for TPU’s on datacenter scale, up to 7x speedup in training. As people begin developing customized AI-hardware, there will be a dance between optimizing new hardware for existing AI models and optimizing new AI models for existing hardware. Google, for better or worst, is well-posed to do both
📱Also, apparently Google’s Pixel smartphone can automatically call emergency services in the event of a car crash
💸[VentureBeat] A nice overview of the difficulties in building GPT-3 business models
💻 A great slide-deck by Amir Gholami on the future of AI compute, delivered as the opening keynote at Intel System Architecture Summit.
Keeping up with the transformers: a new arxiv preprint demonstrates the first all-transformer GAN, “Two Transformers make one strong GAN”

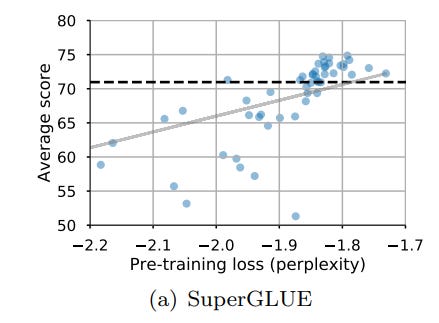
Near-Future Science
🌎[Arxiv] “Integrating Machine Learning for Planetary Science: Perspectives for the Next Decade” - a particularly striking figure of the amount of ML incorporated into NASA research
🪐[IEEE Spectrum] The story of NASA’s perseverance (running on open-sourced Linux!)
AI Feynman: using symbolic solvers and deep learning to derive 100 equations from the Feynman Lectures on Physics [paper][code][data]
[Nature Communications] Converting patent data into experimental synthesis procedures. Most of the worlds latent scientific knowledge is locked in people’s brains or in pdf’s - NLP is helping to unlock these pools of knowledge
Tales of the Twitterverse:



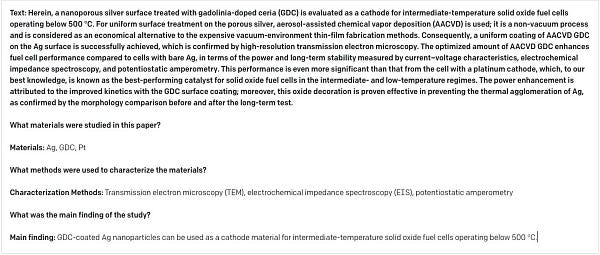

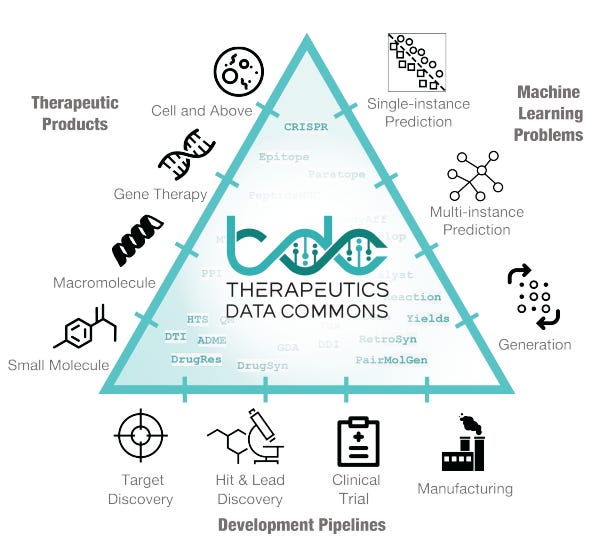
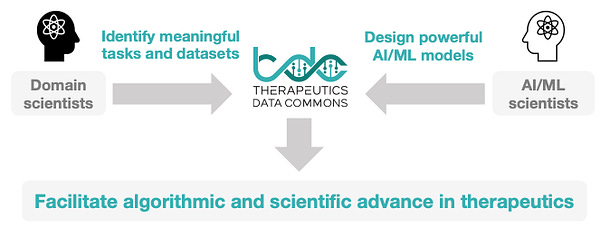
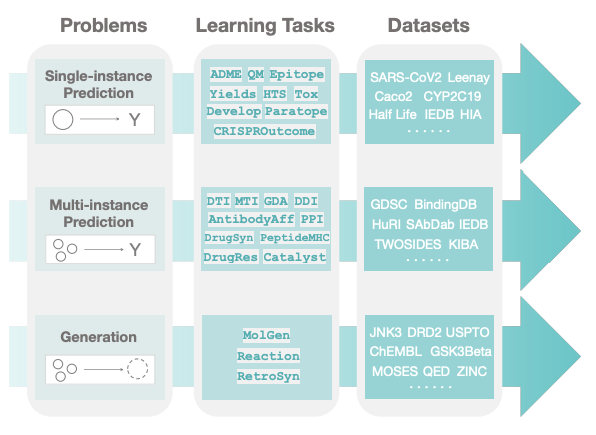
The Science of Science
An actually useful application of blockchain, from Packy McCormick’s Substack (NFT = non-fungible token, of which blockchain is a prominent example)
Matt Clancy dives into how innovative collaborations occur: “Adjacent knowledge is useful”. For instance, an examination of agricultural patents shows most of them cite adjacent knowledge, as opposed to topics that are directly related.
ML4Sci is predictated on the hypothesis that AI is going to become a knowledge multiplier lying within the adjacent possible of every scientific field
[Reddit] “A good title is all you need” - why are preprints on Arxiv popping up with increasingly catchy, but descriptively useless, titles?
🌎Out in the World of Tech
🎲[TechCrunch] Latitude, an AI startup built around creating inifinite game narratives, raises a $3.3M seed fund. Also, Latitude posted a job-posting for GPT-3 hackerer: are these the new jobs promised by the AI revolution?
[Reuters] “Google fires second AI ethics leader as dispute over research, diversity grows”
Policy and Regulation
💰[CSET@Georgetown] A nice breakdown of corporate investors in AI startups based on Crunchbase data. A mix of tech companies (Google, Intel) and finance (Wells Fargo, Goldman Sachs) top the list.
🤐[Protocol] “I helped build ByteDance's censorship machine” - the dark side of TikTok’s parent company in China
Thanks for Reading!
I hope you’re as excited as I am about the future of machine learning for solving exciting problems in science. You can find the archive of all past issues here and click here to subscribe to the newsletter.
Have any questions, feedback, or suggestions for articles? Contact me at ml4science@gmail.com or on Twitter @charlesxjyang