ML4Sci #5: High Throughput-experimentation and AI for optical property prediction; Predicting Cardiovascular health from retinal scans; AI, COVID-19, and Society
Hi, I’m Charles Yang and I’m sharing (roughly) monthly issues about applications of artificial intelligence and machine learning to problems of interest for scientists and engineers.
If you enjoy reading ML4Sci, please hit the ❤️ button above. Or forward it to someone who you think might enjoy reading it!
ML for optical properties
A lot of interesting work is appearing in mapping different forms of spectroscopy to each other. You can imagine one way of measuring a material’s property might be correlated with another property e.g. color with band gap, XRF to film thickness, etc. and some forms of spectroscopy may be more accessible, cheaper, faster, etc. In this work, researchers from the Joint Center for Artificial Photosynthesis (JCAP) at Caltech develop a mapping between RGB images to absorption spectra of metal oxides. What makes this work so interesting is that they use high-throughput experimental data of “178,994 distinct materials samples spans 78 distinct composition spaces, includes 45 elements, and contains more than 80,000 unique quinary oxide and 67,000 unique quaternary oxide compositions” (and open sourced too).
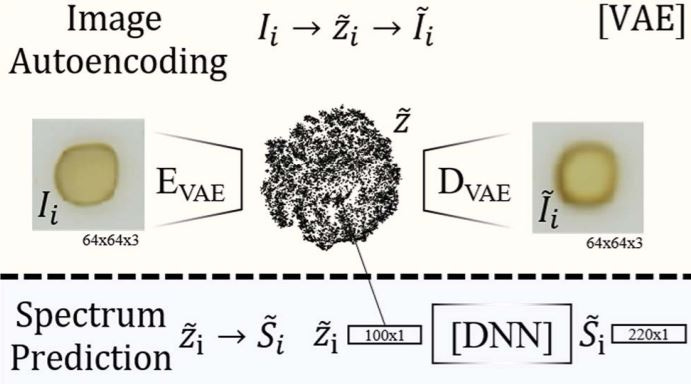
Using a variational autoencoder (VAE), they developed a latent variable representation of the optical images of these materials. The latent variables were passed as input to train a separate neural network to predict the absorption spectra. One of the key highlights of this work is that by using the predicted absorption spectra, they derived a predicted band gap and compared it to a separate algorithm used to extract band gaps from optical spectra. The mean prediction error was 0.18 eV, which is much smaller than the prediction error of band gap by ab-initio DFT methods (which can be up to 1 eV).
The authors extend this work beyond simply using VAE to efficiently train a neural network to map between optical image and absorption spectra, but also use a conditional VAE (cVAE) to “dream up” optical images of new materials based on a target absorption spectra. In addition, the authors open-source their dataset so you can take a lot at their data and try to reproduce their results.
👁️Predicting cardiovascular risk from retinal scans
Another work from Google Research and Verily Life Sciences (a subsidiary of Alphabet, Google’s parent company), in partnership with Stanford School of Medicine. As I’ve been reiterating throughout this newsletter, private companies (mostly Google) have been using their ad-based profits to invest in AI+science fields. Only time will tell how the privatization of the intersection of ML4Sci will affect science as a public resource. Data siloing, particularly in the mostly privatized healthcare space in the US, and it’s colocation with AI expertise/compute resources/domain expertise, will affect who is able to do this kind of research.
In previous work, the authors used Retinal Fundus Photographs to predict Diabetic Retinopathy. Importantly, there is a known and clear medical link between these Retinal Fundus photographs, which are taken at your regular optometrist visit, and diabetic retinopathy, a diabetic complication that can eventually lead to blindness. The data labels are provided by optometrists who manually examine each photograph.
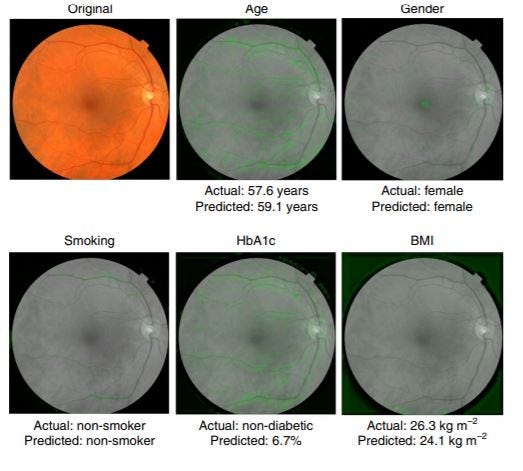
In this current work, they were able to use these diabetic retinopathy images to predict seemingly unrelated characteristics, like age, gender, and various cardiovascular risk factors. They extracted saliency maps from trained NN’s to examine what the model was “looking at” in the image to determine these features. Deep learning is able to tease out correlations with features that human scientists might never think to look for in vast amounts of data. On the other hand, this has implications for how the vast amounts of data we surrender can be de-anonymized by deep learning biometrics (I wonder how many of these patients, who presumably agreed to release this data, knew their age, gender, smoking habits, BMI, etc. could also be calculated). The use of large-scale data mining that sometimes results in unexpected de-anonymization is an increasingly common phenomena, ranging from the netflix challenge to using heart beats and gait for biometrics.
[pdf][Nature Editorial][Google blog]
COVID-19 and Technology
COVID-19 is now officially a global pandemic - it will certainly not go away any time soon. The death toll has continued to rise around the world and doubtless will continue to as various western countries begin fighting to keep the virus under control. Regardless of where you are in the world, continue to wash your hands frequently and avoid touching your face. One important side-story is how our response to Coronavirus, and how we fight it, has changed greatly due to different technological trends. Here are some of the interesting readings I’ve found about how technology, science, AI, and Coronavirus intersect.
Google’s DeepMind open-sources predictions of protein structures associated with COVID-19 [check out our coverage of AlphaFold in ML4Sci #3]
American Physical Society (APS) cancels their conference 36-hours before it starts due to Corona virus fears (as did the American Chemical Society)
Continuously updated news series for COVID-19 by Nature
How preprint servers and Slack are changing the way researchers fight infectious diseases
How China’s Big Data collection on it’s citizen’s helped them contain Coronavirus
Azeem Azhar’s Exponential View has some good insights on how technology and society are being shaped by this new epidemic: 6 ways coronavirus will change our world, after the virus.
It’s also easy for some (namely Silicon Valley techies) to think that technology can solve every problem, including COVID-19. While technologies such as Zoom have made it easier to work from home and advancing drug diagnostics have accelerated testing, there is still no substitute for good testing, unified public messaging, and prepared medical systems (all of which the are lacking in the US response). If you haven’t seen this graphic already, the Economist has a great graphic on how “flattening the curve” makes an epidemic manageable for a country’s healthcare system.
Do you have any articles to share or thoughts on how Coronavirus is teasing out different societal trends? Feel free to leave a 💬 on what you think!
Thank You for Reading!
I hope you’re as excited as I am about how we can use machine learning to solve exciting problems in science. You can find the archive of all past issues here and click here to subscribe to the newsletter.
Have any questions, feedback, or suggestions for articles? Contact me at ml4science@gmail.com or on Twitter @charlesxjyang21