ML4Sci #22: Why I love Random Forests; AI as Software; Podcasts
A more personal edition of (some) of the things I do
Hi, I’m Charles Yang and I’m sharing (roughly) weekly issues about applications of artificial intelligence and machine learning to problems of interest for scientists and engineers.
If you enjoy reading ML4Sci, send us a ❤️. Or forward it to someone who you think might enjoy it!
As COVID-19 continues to spread, let’s all do our part to help protect those who are most vulnerable to this epidemic. Wash your hands frequently (maybe after reading this?), wear a mask, check in on someone (potentially virtually), and continue to practice social distancing.
I want to take this issue as the opportunity to talk a little bit about the work that I actually spend my “day-job” on, because 1) I like sharing about the things I work on and 2) I think its important to be up-front about the contours of one’s personal knowledge. Hopefully this will give you a good idea of the kinds of things I’m exposed to on a daily basis.
🎙️First, some housekeeping. I had the chance to talk with Charlie You on his ML Engineered Podcast about how AI is changing the way we do science, writing this newsletter, and how my faith motivates my work (starting at 1:09:25). Check it out if you like podcasts or machine learning!
🚪Awhile ago, I wrote about some new work I’ve started on backdoor models. This past week, I wrote a Medium article titled “AI is a cybersecurity threat” about why we need to start thinking about deep learning models as pieces of software, with all the associated set of cybersecurity flaws and vulnerabilities. What we currently lack is the ability to interrogate models and discover if they have flaws and that’s a problem (this perspective of models as software is also useful when thinking about productionizing models within larger software ecosystems).
Finally, here are two papers I co-authored this past year, which train and interpret Random Forest models for totally different applications in totally different ways
Vibrational detection of delamination in composites using a combined FEM and ML approach
Composites, particularly 3D-printed composites, are increasingly being used in safety-critical applications like aerospace and aviation. To ensure high-quality and high-throughput manufacturing and safety validation, we need simple and non-detective characterization methods to look for delaminations in composites. For instance, if SpaceX wants to build reusable rockets, then they need to be able to check the structural quality of the rocket after each flight without destroying it.
In this work, we consider 3 different (and increasingly complex) non-destructive methods of characterizing composites and examine their ability to reveal the presence of delaminates.
We also use Random Forests to localize delaminates using data from different characterization methods. This would be the simple get-data-then-model-fit part of the project. But the most exciting part is then we apply a simple approach to convert any prediction by the random forest into a linear equation. When we examine the model weights with respect to the simulated delaminate size, we find there is a startlingly stark “phase transition” in model weights for higher-order mode curvatures. When we examine the simulated dataset, we find that this phase transition in model weights actually corresponds to a physical phenomena, for which we currently do not have an explanation.
The below figure illustrates the phase transition for higher order mode curvatures (a) and the lack of a phase transition in model weight for lower order modes (b). This model-based phenomena is reflected in the simulations of physical behavior shown below for each mode.
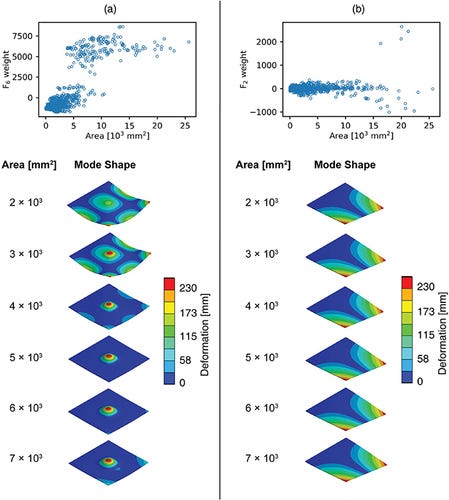
In other words, we use a simple interpretability method for Random Forests. On one of the composite delamination tests, we find a change in model weights which turns out to corresponds to an actual physical phenomena. In other words, by probing our model, we are able to discover something hidden in the data that we would not looked for previously (and for which we currently lack an explanation)!
[paper] this link will be paywalled starting 1 week after this issue goes out - email me for me a pdf
Interpretable inverse design of particle spectral emissivity using machine learning
In this paper I did as part of my work at Lawrence Berkeley National Lab, we set out to perform inverse design of optical metamaterial properties for particle emissivity. First, we apply the standard get-data-then-model-fit approach with a Random Forest on a dataset of different geometric particles, with 3 different types of materials, over a wide variety of length scales.
Now for the interesting part: we leverage the fact that it is basically free for us to generate particle geometries (e.g. inputs). The computationally expensive part is mapping input (particle) to output (spectral emissivity). This is not normally true in most computer vision problems (scraping faces i.e. inputs, still takes effort). We generate orders of magnitude more particle geometries than originally available and use the trained Random Forest as an emulator for an optical solver. Finally, we train a decision tree on this massive, random-forest-labelled dataset and use it to perform inverse design by doing leaf-lookups. In other words, given a desired optical emissivity spectra, the decision tree trained on the massive random-forest-labelled dataset will return a set of design rules for geometries that will give the desired spectra.
In the below figure, we show how our decision tree (DTGEN) has enhanced inverse design compared to decision trees naively trained on the original dataset. Notice also how we are able to generate high optical emissivity samples via inverse design despite the vast majority of the training data being biased to low emissivity (histograms display integrated emissivity as calculated by a physics optical solver).
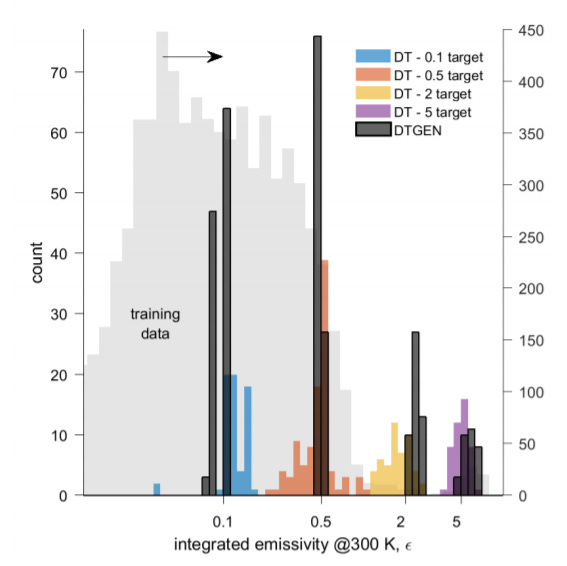
This paper is a good example of how scientific problems have different constraints and data environments than standard ML tech problems. We leverage the fact that we are able to generate parameterized inputs for free and base our work on the importance of interpretability in inverse design.
[paper]
Some meta points:
Astute readers will notice that despite how much I harp on open-science and open-data, I have not provided any code or data for my own papers! Unfortunately, despite my personal opinion, I am working in an ecosystem that, as I have discussed, lacks proper incentive structures to encourage open-data and unfortunately, I am not the sole stakeholder on these projects. With that said, I believe we will be releasing the code and data for “interpretable inverse design…” once it is published (we’re currently wrapping up peer review).
The first paper essentially took 5 months from project conception to publication; we followed a fairly linear, waterfall type project progression. The random forest interpretability was a nice surprise at the end for us, but everything else was fairly “expected”. The second paper took over a year, is totally different from the initial project conception, and is still not published yet (currently wrapping up peer review). That’s science I guess? Imagine if we actually had to do real experiments too 🙄
If you can’t tell, I really like Random Forests (and their hipper, younger, cousins, lightgbm and xgboost). They work out of the box, give excellent performance on tabular data, and there are a ton of tricks you can use to get interpretability or explanations out of them.
One of the core hypotheses of this newsletter is that scientific problems live in a different space than most tech industry problems, which means that we can do different things with our models (and are worried about different things). I hope its clear now why I hold that opinion so strongly, given all the ways its cropped up in my work.
And now, for the news.
📰In the News
ML
blog-of-the-week: a massively detailed post on which GPU to get
“It’s not just that size matters: Small Language Models Are Also Few-Shot Learners”
Cute name aside, this work shows that we can get GPT-3 performance on a model with 0.1% as many parameters
“Science is a verb: adopting the scientific method and best practices in AI research”
A great talk from Michela Paganini
🎰Must-read: Hardware lottery
“This essay introduces the term hardware lottery to describe when a research idea wins because it is compatible with available software and hardware and not because the idea is superior to alternative research directions.”
Science
🎲Stirring 25,000 dice leads to crystalline order[APS Physics]
Crystals.ai - a cool website of different materials science AI models and datasets hosted by the Materials Virtual Lab at UC San Diego
🔥Smoke Has Caused Temperature Forecasts to go crazy - how a blindspot in weather models caused temperature forecasts to be way tooo high while the west coast is on fire. I wonder what kind of blind spots AI weather models will have 🤔
The Science of Science
Congrats to SciML for being added to the cohort of NumFOCUS sponsored projects [h/t Chris Rackauckas]
A novel approach to predicting exceptional growth in research[PlosOne][data] - exciting new work that aims to predict exceptional growth in different research fields. See below for their 2018 forecast for fields in computing technologies (notice the dominance of US, China, and Alphabet, Google’s parent company)
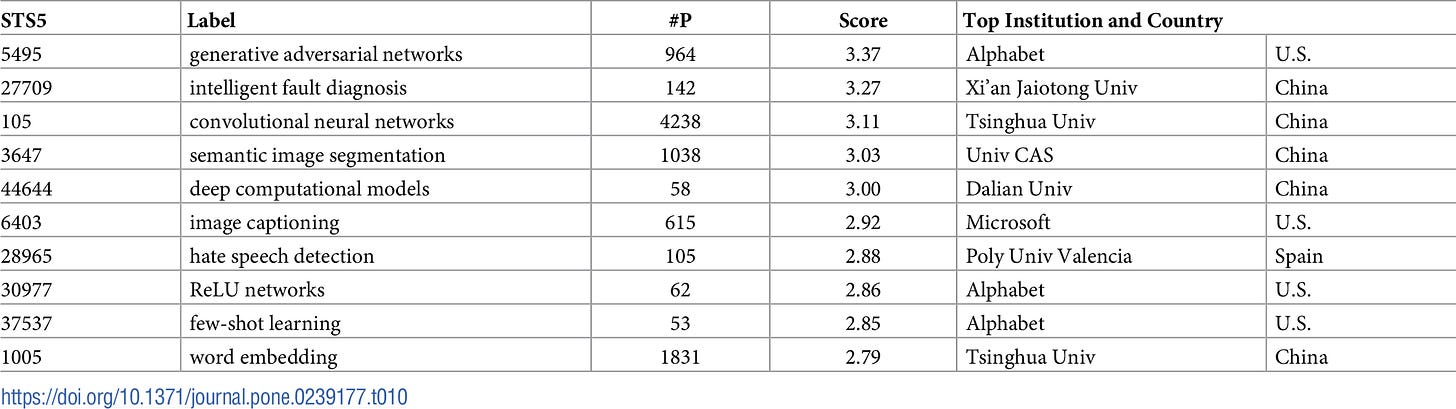
📈Forecasting Timelines in Quantum Computing
From the abstract:
“…we estimate that that proof-of-concept fault-tolerant computation based on superconductor technology is unlikely (<5% confidence) to be exhibited before 2026, and that quantum devices capable of factoring RSA-2048 are unlikely (<5% confidence) to exist before 2039.”
📚“Science is getting harder to read”[NatureIndex]. Complexity increasing, abstraction rising - peer review is not the right regularization for making scientific writing clearer (clear writing is also hard in general)….maybe we need newsletters?
The open-access rebellion continues[Twitter]:


🌎Out in the World of Tech
🌊Microsoft dumps a cloud server underwater and…it worked out pretty well
NVIDIA buys ARM - big implications as the world’s largest GPU’s maker buys the chip company that has its designs in 95% of mobile devices
Microsoft gains exclusive license to use OpenAI’s GPT-3 model
See more: “AI Democratization in the Era of GPT-3”[Gradient]
Policy and Regulation
“Scientists use big data to sway elections and predict riots — welcome to the 1960s”[Nature Commentary] or, “why we need to teach computer scientists history”
🇺🇸 CSET publishes AI policy recommendations for the next US presidential administration
Also, “Strategic Re-engineering” - rebuilding US R&D
Thanks for Reading!
I hope you’re as excited as I am about the future of machine learning for solving exciting problems in science. You can find the archive of all past issues here and click here to subscribe to the newsletter.
Have any questions, feedback, or suggestions for articles? Contact me at ml4science@gmail.com or on Twitter @charlesxjyang