ML4Sci #20: Discovering Symbolic Models from Deep Learning with Inductive Biases; Inverse Design of Crystals using Generalized Invertible Crystallographic Representation
Also, a primer on backdoor models and robustness
Hi, I’m Charles Yang and I’m sharing (roughly) weekly issues about applications of artificial intelligence and machine learning to problems of interest for scientists and engineers.
If you enjoy reading ML4Sci, send us a ❤️. Or forward it to someone who you think might enjoy it!
As COVID-19 continues to spread, let’s all do our part to help protect those who are most vulnerable to this epidemic. Wash your hands frequently (maybe after reading this?), wear a mask, check in on someone (potentially virtually), and continue to practice social distancing.
Discovering Symbolic Models from Deep Learning with Inductive Biases
Published June 19, 2020
“Mathematics is the language in which God has written the universe”
- Galileo Galilei
This new work from Cranmer et. al. uses graph neural networks combined with a traditional symbolic solver to propose a new analytical equation for dark matter interactions in a gravitational simulation of the Universe, which outperforms previously constructed heuristics. The model was also validated on newtonian and hamiltonian models and demonstrates excellent performance both in terms of predictive accuracy and analytical formulation. The authors also demonstrate how their model can have an inductive bias towards lower-dimensionality representations via regularization and is also flexible enough to incorporate different formulations e.g. hamiltonian, lagrangian.
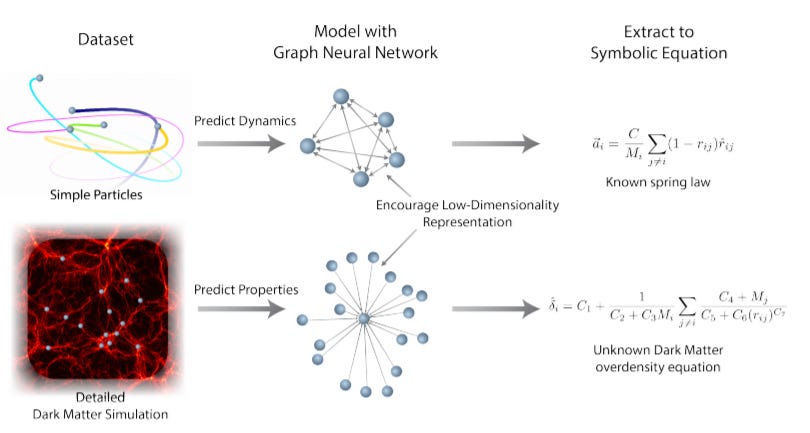
See also:
A new preprint of a graph representational learning textbook from McGill University
I’ve also previously covered graph neural networks in glassy systems, quantum chemistry, and for discovering new antibiotics
Inverse Design of Crystals using Generalized Invertible Crystallographic Representation
Published June 22, 2020
This international collaborative work develops invertible 2D representations of 3D crystals based on data from the Materials Project. A Variational Autoencoder (VAE) is used to propose new crystal structures not in the original Materials Project Database. These predictions are then validated with Density Functional Theory (DFT).
To featurize 3D representations, this work uses both the real-space and momentum-space representation of a 3D crystal structure. The VAE architecture is shown below - it is trained on data from the Materials project in a forward pass and for inverse design, a trained NN is used to identify samples from the latent space with favorable properties, and the decoder is used to map to the actual crystal structure.
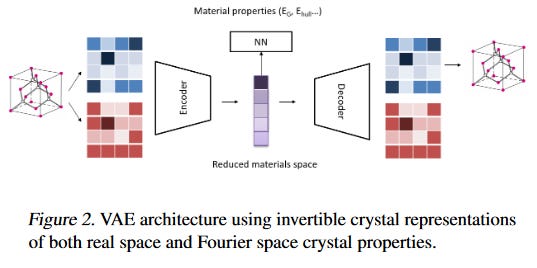
Finally, the authors demonstrate their model has predictive accuracy within the margin of error of DFT and use it to perform inverse design of thermoelectric materials, generating high-performance crystal structures not in the Materials Project Database. 2 out of the novel 27 proposed crystal structures show state-of-the-art thermoelectric performance. The authors point out that future work remains (in the rest of material science as a whole as well) as for how to elucidate synthesis procedures for novel structures designed by machine learning methods.
See also:
Materials Project Website - the director of Materials Project, Kristin Persson, was also recently announced as the new director of the Molecular Foundry at Lawrence Berkeley National Lab
Position and Momentum Space i.e. real and fourier space [Wikipedia]
[paper]
🚪Backdoor Learning: A Survey
Published July 17, 2020
I’ve recently started looking into backdoor learning for a new research competition I’m joining hosted by NIST and IARPA(basically DARPA but for AI). It’s not strictly related to ML4Sci, but it does help highlight the non-intuitive weakness of neural networks and may provide insight into how to build robust and generalizable models (it’s also, in my opinion, just pretty cool).
For context, it has been known for quite some time that neural networks are quite fragile and can be tricked into misclassifying inputs by applying a small amount of noise, which are known as adversarial examples. This is an inference-side attack: given a seemingly well-trained model, an adversarial user can create inputs that the trained model misclassifies. See the below figure from “Explaining and Harnessing Adversarial Examples” by Goodfellow, Shlens, and Szegedy.
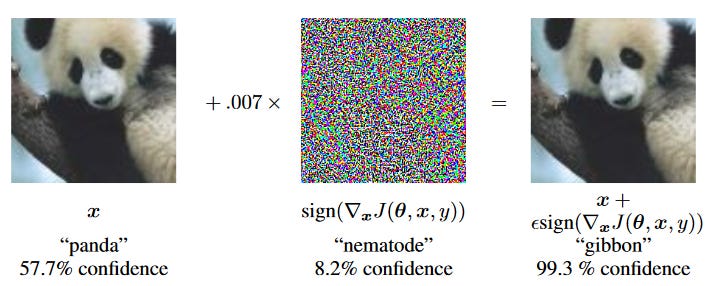
Backdoor attacks on the other hand assume a malicious user is the one training the model. The motivating problem is based on the observation that in many fields, we rely on massive pre-trained models, usually built by private companies, which are then fine-tuned locally by a user e.g. ResNet, BERT, OpenAI’s GPT-3. But what if these massive pre-trained models have a built-in backdoor? For instance, Tesla might download an ImageNet model and then fine-tune it to use in a self-driving car. But what if the massive ImageNet model has a backdoor built into it that, when it sees a particular sticker on a stop sign, causes it to misclassify it?
This is different than adversarial training, because in that threat model, we assume a malicious user is trying to break a model e.g. cause misclassification. In backdoor learning, we assume that the model itself has a backdoor built into it by a malicious actor, and the user who deploys it has no idea that it exists.
We used to think of deep learning models as just that: models. But their massive overparameterization and brittleness means they contain security vulnerabilities analogous to that of traditional software. The challenge is that unlike with traditional software, which theoretically can be verified by independent experts, we currently have no idea how to tell if a deep learning model has a backdoor in it! (and this is the problem I’m working on for part of my research)
See also:
Breaking Tesla Autopilot with Stickers[from Tencent]
[survey]
📰In the News
ML
“Reflecting on a year of making machine learning actually useful” Another one of those great blogs strewn across the field of the internet that demonstrates what ML is actually like in the real world (hint: mostly fixing SQL joins)
🧠Does a grandmother cell a.k.a. Jennifer Aniston neuron exist? Experiments with neural networks suggest no, with implications for interpretability[Google AI]
AWS releases CodeGuru, which claims to provide ML-powered automated code review
Science
🦠Recursion releases 3 massive cell imaging datasets
Facebook AI Research (FAIR) publishes new work that accelerates time to complete MRI scan by factor of 4 [FAIR Blog] Good example of 1) how AI can improve our ability to measure things, which will have an unknown cascade of positive effects, not just in healthcare, but in material science, physics, astronomy, etc. 2) tech companies like Facebook are driving AI-based research in a variety of seemingly disparate fields
📚Jupyter announces “Jupyter Book'“: embedding executable code as book formats. Imagine a world where you can buy digital textbooks with interactive python code for simulations and plotting built in!
QuantaMagazine releases an interactive map of the “Theories of Everything”. You can find their previously released interactive Map of Math here.
From Biorxiv: Learning with uncertainty for biological discovery and design. A good example of why uncertainty estimates are so important in science, and why gaussian processes are still alive and kicking in the sciences, despite the deep learning craze. From the abstract:
By leveraging Gaussian process-based uncertainty prediction on modern pretrained features, we train a model on just 72 compounds to make predictions over a 10,833-compound library, identifying and experimentally validating compounds with nanomolar affinity for diverse kinases and whole-cell growth inhibition of Mycobacterium tuberculosis
“From Desktop to Benchtop – A Paradigm Shift in Asymmetric Synthesis” Also in Nature Catalysis🔒 From the abstract:
The organic chemist’s toolbox is vast with technologies to accelerate the synthesis of novel chemical matter. The field of asymmetric catalysis is one approach to access new areas of chemical space and computational power is today sufficient to assist in this exploration. Unfortunately, existing techniques generally require computational expertise and are therefore under-utilized in synthetic chemistry. We present herein our platform Virtual Chemist that allows bench chemists to predict outcomes of asymmetric chemical reactions ahead of testing in the lab, in just a few clicks
For more on automation, AI, and science - see also:
ML4Sci #15: Bayesian Autonomous Experimental Researcher for 3D printing
Also, time-lapse of the printing process for the world’s largest 3D printed boat
The Science of Science
“Too many AI researchers think real-world problems are not relevant”[MIT Tech Review] How biases in what reviewers consider relevant problems at top AI conferences is holding back the field from publishing research on concrete implementations of AI.
🧐A Computational Lens on Economics[ACM] One of many examples of how different perspectives can enable new insight
From Google AI: An Analysis of Online Datasets Using Dataset Search - a survey of how people publish datasets
🌎Out in the World of Tech
DARPA wraps up Alpha Dogfight AI Fighter Pilot Challenge: AI defeats human pilot in simulations 5-0 [DefenseOne] [Video]
Impact of Go AI on the professional Go world. Some similarities, some differences with how AI affected the chess world
🚚Google’s Waymo Tests Autonomous Trucks in Texas
Inside the Hidden World of Legacy IT Systems - important because AI software today is built on top of modern frameworks like Pytorch and requires large amounts of high-quality data. I would argue that lots of industries have the potential to be disrupted by newer startups with more modern software stacks, which allows for easier integration of AI into the software stack.
Policy and Regulation
US establishes federal AI and quantum computing research centers
The AI triad[CSET] c.f. nuclear triad
Thanks for Reading!
I hope you’re as excited as I am about the future of machine learning for solving exciting problems in science. You can find the archive of all past issues here and click here to subscribe to the newsletter.
Have any questions, feedback, or suggestions for articles? Contact me at ml4science@gmail.com or on Twitter @charlesxjyang