ML4Sci #14: Uncertainty Quantification using NNs for Molecular Property Prediction; SunDown: model-driven per-panel solar anomaly detection
In the News: COVID-19 & science, OpenAI GPT-3's new API, and how to write rebuttals to reviewer comments
Hi, I’m Charles Yang and I’m sharing (roughly) weekly issues about applications of artificial intelligence and machine learning to problems of interest for scientists and engineers.
If you enjoy reading ML4Sci, send us a ❤️. Or forward it to someone who you think might enjoy it!
As COVID-19 continues to spread, let’s all do our part to help protect those who are most vulnerable to this epidemic. Wash your hands frequently (maybe after reading this?), check in on someone (potentially virtually), and continue to practice social distancing.
Uncertainty Quantification using Neural Networks for Molecular Property Prediction
Published May 20, 2020
As I’ve discussed in ML4Sci #9, the field of computer vision may be overfitting to standard ML benchmarks, like MNIST, CIFAR-10, and ImageNet. Different training tricks or new architectures may claim to offer improvements on these datasets, but after a decade of optimizing for the same set of datasets, one may wonder if the entire field hasn’t “overfit”. Conveniantly, ML4Sci offers a wealth of broad datasets that can serve as a good validation set for certain ML methods that claim to be generalizable.
In this work, EECS and chemical engineering researchers team up to evaluate a gamut of uncertainty quantification (UQ) methods for neural networks on a set of molecular datasets. UQ is of great interest for scientists, because we would like to know how confident a model is in its predictions, in order to inform human decision-making. They test the following UQ methods on both message-passing neural networks (MPNN), which we covered in ML4Sci #6, and feed forward (FF) neural networks:
ensembling (traditional, bootstrapping, snapshot, MC Dropout)
Mean and variance estimation
Distance Based methods (latent, structure space)
Union based methods (Gaussian, RF), which are trained on latent space representations
Using a variety of metrics to assess UQ performance on a variety of datasets, the authors find that there is wide variance between different UQ model performances across different datasets. In other words, there is no “silver bullet” UQ method that works well on all datasets. Some general trends observed are that MPNN’s generally outperform FF neural networks and that union-based methods, which train traditional ML models like random forests on the latent space representation, tend to perform well.
This paper is a good demonstration of a hypothesis readers of the newsletter are familiar with: ML4Sci is a two-way street and ML practioners can learn or use ideas from the physical sciences, in the same way scientists are making use of recent AI advances. In this case, this paper is a good demonstration that ML4Sci data may be useful for ML model development and benchmarking.
SunDown: model-driven per-panel solar anomaly detection for residential arrays
Published on May 25, 2020
Improving the efficiency of residential solar arrays will help drive adoption and lower cost. This paper showcases a “sensorless approach designed to detect per-panel faults in residential solar arrays” by using “a model-driven approach that leverages correlations between the power produced by adjacent panels to detect deviations from expected behavior”. The model achieves >95% accuracy at classifying the underlying cause of both single-panel and and concurrent faults.
Anomalies studied include occlusion from dust, snow, or leaves, and power faults. Two datasets are used: one is from a real-world roof installation with hand-labelled snow occlusion, the other is from a ground-mounted test installation with artificial tests done, as shown below. The intuition behind the modelling is that the arrays are all dependent on each other and that a given arrays power output can be predicted by examining the power output of the arrays next to it. A simple bayesian model is used that bootstraps a subset of the other panels to determine if a given panel has a fault/occlusion. They also generalize this method to detect multiple concurrent faults. This paper is a cool illustration of how fairly simple ML ideas can be used to lower cost and improve efficiency of important real-world systems, like residential solar arrays!
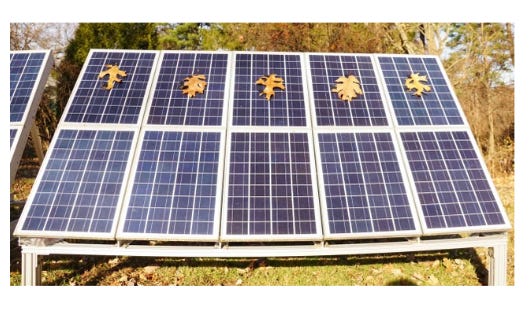
[paper]
📰In the News
ML
From Google/DeepMind. Acme: A new framework for distributed reinforcement learning[blog][code][paper]
Eye-catching advances in some AI fields are not real [Science Mag]
Last week, OpenAI released GPT-3, a massive 175B NLP model. This week, they are releasing a preliminary API for companies to use (as opposed to open-sourcing the model itself)[blog post]. It’s additionally interesting because of how OpenAI has changed from a non-profit to a “capped profit” - this API could end up being quite lucrative for them.
Towards the systematic reporting of the energy and carbon footprints of machine learning
Science
A mysterious company’s coronavirus papers in top medical journals may be unraveling[ScienceMag]. “Here we are in the middle of a pandemic with hundreds of thousands of deaths, and the two most prestigious medical journals have failed us”. The studies have now been retracted.
The Science of Science
How to write rebuttals to reviewer comments
MIT, joining the UC system, also cancels contract with publishing giant Elsevier
Will the pandemic permanently alter scientific publishing? [Nature News]
🌎Out in the World
Zoom closed US accounts of chinese users protesting the 1989 Tiananmen square protests.
Another one from CSET: AI Hubs in the USA and Chinese Investment
IBM, Amazon, Microsoft, halt facial recognition programs in response to concerns over police brutality and racial inequality
Thanks for Reading!
I hope you’re as excited as I am about the future of machine learning for solving exciting problems in science. You can find the archive of all past issues here and click here to subscribe to the newsletter.
Have any questions, feedback, or suggestions for articles? Contact me at ml4science@gmail.com or on Twitter @charlesxjyang