ML4Sci #19: Pushing the limit of MD with ab-initio accuracy to 100 million atoms with ML; Randomized Automatic Differentiation
Also, another COVID-19 news roundup
Hi, I’m Charles Yang and I’m sharing (roughly) weekly issues about applications of artificial intelligence and machine learning to problems of interest for scientists and engineers.
If you enjoy reading ML4Sci, send us a ❤️. Or forward it to someone who you think might enjoy it!
As COVID-19 continues to spread, let’s all do our part to help protect those who are most vulnerable to this epidemic. Wash your hands frequently (maybe after reading this?), wear a mask, check in on someone (potentially virtually), and continue to practice social distancing.
Just to make what has already been happening unofficially now official: I’ll be transitioning to a slightly different format. Instead of an in-depth focus on a few papers, I’ll be transitioning to a slightly broader overview of multiple papers, as well as an expanded commentary on what’s happening in the news (now with another subsection on policy and regulation).
Working at the intersection of ML and Science requires a broad understanding of trends in both of these fields, the unique properties that emerge at their convergence, and how external factors, like government regulations and investment capital, affect them - I’m still trying to figure out how to balance this in the newsletter coverage. As always, I’m more than happy to take feedback or suggestions for articles. Feel free to drop a comment 💬, contact me at ml4science@gmail.com, or on Twitter @charlesxjyang21
Pushing the limit of molecular dynamics with ab-initio accuracy to 100 million atoms with machine learning
Published May 1, 2020
In this time of international political strife, and one that often catches researchers in the crossfire, it is worth noting that this work was published by both US and Chinese researchers and ran on the Summit Supercomputer at Oak Ridge National Lab.
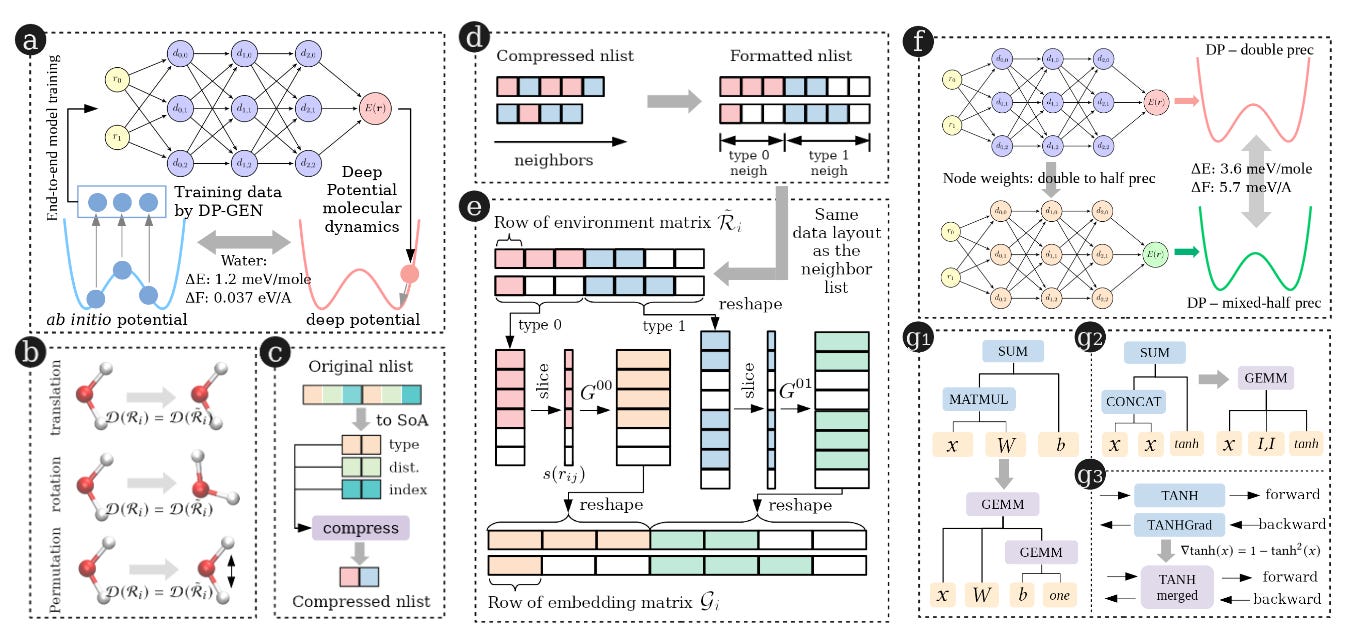
Efficiently scaling up modelling paradigms to supercomputer scales, especially when we run software on heterogeneous hardware types (CPU’s and GPU’s), is a challenge in and of itself. This work uses Deep Potential Molecular Dynamics (DeePMD) to predict the nanosecond-long dynamics (with femtosecond resolution) of 100 million atom systems using a variety of optimizations to significantly improve efficiency on the Summit supercomputer, including mixed-precision computations, replacing Tensorflow Matrix-Multiply calls with CUBLAS GEMM, and optimizing the flow of data between different parts of the supercomputer. They demonstrate their model’s near-linear scaling on the Summit supercomputer on both water and copper simulations with millions of atoms, enabling simulations of nanoscale time lengths in under 2 hours.
The real utility of ML4Sci will show when we replace supercomputers traditionally used to run numerical simulations (e.g. weather simulations), with deep-learning enabled models trained on massive datasets; this paper shows both the difficulties and paths forward in getting there.
For other coverage of the Summit supercomputer, see ML4Sci #7: Exascale Deep Learning for Scientific Inverse Problems
[paper]
Randomized Automatic Differentiation
Published July 20, 2020
Common neural network implementation packages like Keras, Pytorch, and Tensorflow build a computational graph of mathematical operations, with a known set of derivatives, and use these in-built functions to automate gradient backpropagation. And it is this gradient backpropagation, that is used to update weights of a neural network, that lies at the heart of deep learning, but is also the largest memory sink. However, we know that stochastic gradient descent is, well, stochastic. In light of this, the authors here ask the following motivating question:
Why spend resources on exact gradients when we’re going to use stochastic optimization?
The authors introduce randomized automatic differentiation, which stochastically samples gradients over a computational graph, rather than explicitly calculating them. They demonstrate significant memory savings for both neural network training and for optimizing linear PDE’s.
COVID-19 Roundup
Estimating the Fatality Rate of COVID-19 is difficult [Berkeley AI Research Blog]. A real-world example of how a plethora of sampling biases leads to “garbage in, garbage out”s
🧬Google’s DeepMind updates on AlphaFold: an experimental group from UC Berkeley mostly confirms AlphaFold’s predictions for a protein
COVID-19’s lingering effects alarms scientists[ScienceMag]
🏠Google will be work-from-home until at least July 2021[TheGuardian]. “Atlassian tells employees they can work from home forever”[CNBC]
GPT-3 Hype
The untold story of GPT-3 is the transformation of OpenAI. The difficulties of building massive AI systems, not making money, and a limited labor pool that is in high-demand
🧠Philosophers respond to OpenAI’s GPT-3
This blog post on how to be productive was trending on Hackernews. It was also (secretly) written by GPT-3. It’s a new world out there[MITTechReview]
📰In the News
ML
🧽Sponge Examples: Energy-Latency Attacks on Neural Networks (crafting adversarial attacks to significantly increase energy consumption or latency e.g. on self-driving cars).
Another excellent blog post by Lillian Weng, this time on Neural Architecture Search. There is a whole universe of excellent blog posts on deep learning spanning from different visualizations for non-math introductions to excellent in-dept technical reviews like these. There’s probably a space somewhere for aggregators of these blog posts, since they’re mostly undiscovered gems strewn across the vast field of the internet.
💊 “Atomwise’s machine learning-based drug discovery service raises $123 million”[TechCrunch]
🔠 NLPprogress tracks progress in different NLP tasks and languages. Resources like these, that track field performance on diverse sets of benchmarks in an open and crowd sourced manner, point to the importance of benchmarks in ML and competition driven fields.
Amazon makes online Machine Learning University open to the public. Remote work+Internet+large tech companies+talent scarcity->making certified education degrees obsolete? Google also has a constellation of educational courses for AI
🚗Autonomous cars: 5 reasons why they still aren’t on the road [TheConversation]
The Carbon Impact of AI[Nature].
Science
📝Domain-Specific Language Model Pretraining for Biomedical Natural Language Processing from Microsoft Research. A new massive dataset of biomedical articles from PubMed
🌽From the Pytorch Medium blog: Production machine learning for agriculture, by startup Blue River Technology, which develops ML-powered weed-spraying machinery. AI-powered Science-as-a-Service Industry 5.0 is here
Deep learning for genomics using Janggu [Nature Communications]. From the abstract:
“Here we present Janggu, a python library facilitates deep learning for genomics applications, aiming to ease data acquisition and model evaluation. Among its key features are special dataset objects, which form a unified and flexible data acquisition and pre-processing framework for genomics data that enables streamlining of future research applications through reusable components. Through a numpy-like interface, these dataset objects are directly compatible with popular deep learning libraries, including keras or pytorch. Janggu offers the possibility to visualize predictions as genomic tracks or by exporting them to the bigWig format as well as utilities for keras-based models. … We believe that Janggu will help to significantly reduce repetitive programming overhead for deep learning applications in genomics, and will enable computational biologists to rapidly assess biological hypotheses.”
The Science of Science
The entire Arxiv repository, 1.7M+ articles, is now available on Kaggle
“The machine learning community has a toxicity problem”[Reddit thread, r/MachineLearning]
UC Berkeley Library article: Will COVID-19 mark the end of scientific publishing as we know it?
A World Without Referees. Some interesting arguments here arguing for disbanding peer review. While I don’t agree with everything here, I think there is value in at least discussing what a better system would look like (as I do here)
Finally, a conference that is standing up to Reviewer #2. See also: “Peer review in NLP: reject-if-not-SOTA”

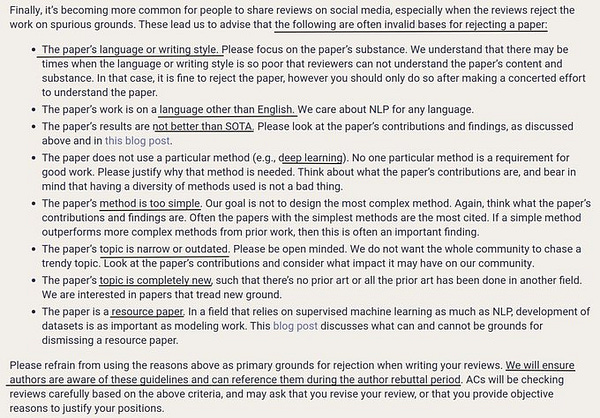
🌎Out in the World of Tech
Using AI to document war crimes[MIT Tech Review]
🛦“The US Air Force is turning old F-16s into pilotless AI-powered fighters”[Wired]
Policy and Regulation
More from Eric Schmidt: “Former Google CEO Eric Schmidt is leading a federal initiative to launch a university that would train a new generation of tech workers for the government”[BusinessInsider]
US General Services Administration (GSA) opens AI challenge with a total of 20K cash prizes for top 3 finalists [github]
🇳🇿The first government to release a whole-of-government approach to using algorithms is…New Zealand
🔒“Explaining” machine learning reveals policy challenges - why “explainable ML” may conflict with the social ambiguities often present in policy making for liberal democracies
Another excellent piece from the CSET think-tank: Deepfakes - A Grounded Threat Assessment
The Humble Task of Implementation is the Key to AI Dominance [WarOnTheRocks]
Thanks for Reading!
I hope you’re as excited as I am about the future of machine learning for solving exciting problems in science. You can find the archive of all past issues here and click here to subscribe to the newsletter.
Have any questions, feedback, or suggestions for articles? Contact me at ml4science@gmail.com or on Twitter @charlesxjyang