ML4Sci #8: Defining the new AI-powered SaaS: Science as a Service
How AI will change science, industry, and manufacturing
Hi, I’m Charles Yang and I’m sharing (roughly) weekly issues about applications of artificial intelligence and machine learning to problems of interest for scientists and engineers.
If you enjoy reading ML4Sci, please hit the ❤️ button above. Or forward it to someone who you think might enjoy reading it!
If someone forwarded this newsletter to you and you want to get one delivered to you every week, you can subscribe to receive this newsletter by clicking below
As COVID-19 continues to spread, let’s all do our part to help protect those who are most vulnerable to this epidemic. Wash your hands frequently (maybe after reading this?), check in on someone (potentially virtually), and continue to practice social distancing.
Defining the new SaaS: Science as a Service
AI is becoming the transformational technology that will define the next wave of a broad swath of industries, similar to the massive online e-commerce shift that began in the late 2000’s. Oftentimes with new technological shifts, one of the most important resulting innovations are new business models. As I’ve been discussing throughout this newsletter, the intersection of AI and science has particular nuances that make its adoption and development subtly different from the development of AI as a whole - the same is true of its business models.
In this essay, I’ll be stepping out of the academic world of ML4Sci and instead examine how AI is shaping the business realm for science-based startups and companies. In some cases, AI is changing the way companies innovate; in other cases, the confluence of other global macro trends, like climate change and IoT, are enabling new AI-powered startups. The thesis of this essay is that we will see a new business model: AI-powered Science as a Service (SaaS).
A16Z, a venture capital firm, has a slogan: “software is eating the world”. The rise of cloud computing and widespread, high-speed internet coverage drove the growth of traditional SaaS enterprises like Google, Zoom, Workday, and Salesforce. By leveraging cloud-hosted servers, SaaS companies are able to sell a software service, rather than a software product. It’s akin to the difference between buying Microsoft Office in the 90’s and using Google Docs today.
Many traditional scientific companies still mostly sell products. But with the rise of AI, software is finally starting to take a bite out of science. We’ve seen in this newsletter how AI-powered models are beating domain experts in protein folding predictions, speeding up scientific simulations, discovering novel antibiotics, and outperforming numerical weather models. While there have been some Science-as-a-Service companies, their dominance has been limited by the complexity of numerical models, the lack of big data and compute infrastructure throughout the sciences and engineering fields, and the insufficient accuracy of numerical models. The convergence of growing open-sourced data, combined with AI, particularly deep learning, has finally resulted in fast and accurate models that generalize to problems of interest to companies. I’ll examine several different types of AI applications, cover startups in different industries, and discuss how they all incorporate Science-as-a-Service business models.
AI for Controls
One situation where AI excels is in controlling complex systems to meet some set of optimization criteria. The popular imagination might jump to self-driving cars, but many important ML4Sci applications falls under this domain as well. There are two particularly exciting fields that fall under this category, with which I am most familiar: renewable energy management and 3D printing.
Renewable energy systems are dynamic: rather than monocultures of power plants, we’ll need diverse systems of wind, solar, hydro and storage to meet demand. These systems all interact with each other and the power grid in complex ways. In particular, optimizing storage systems for variable electricity pricing and battery health is proving to be an important part of the renewable energy solution. Stem is a startup that provides enterprise battery management, while VoltaiQ is a startup that also provides software for battery testing and development. On the customer-facing side, Verdigris and sense provides customers with meter-based insight into how they can cut energy usage. The smart grid raises a lot of interesting optimization applications with potential for integrating AI e.g. dynamic pricing, dynamic load optimization, energy+storage optimization, using EV’s as dynamic storage, etc. with obviously important implications for our environment.
Another complex system with certain desired optimal outputs is 3D printing, which is prone to a variety of dynamic failure modes during printing. As a result, startups like inkbit and Relativity Space are using AI as a controller for 3D printing to ensure high-quality prints. In this case, AI is accelerating the industrialization of another trend, namely 3D printing. Manufacturing and design are typically integrated in a single company because the designers are limited by manufacturing constraints. This is certainly true of rocket engines and yet Relativity Space promises to be able to 3D print rocket engine parts. The ability to disentangle manufacturing and design creates an abstraction barrier that allows companies to offload manufacturing to another company. The dream of 3D printing is to create Manufacturing-as-a-Service and AI is helping to accelerate that goal.
In both of these cases, AI is accelerating the development of external trends. It is making renewable energy more economically cost-effective and 3D printing less prone to defects. However, how AI is integrated in these two industries is different. AI is serving as the inseparable brains of the 3D printer; for now, the 3D printer and the AI controls are inseparable. Yet it is because of AI that 3D printers can serve as a reliable manufacturing service, where designers can submit arbitrarily complex designs for fabrication. On the other hand, VoltaiQ and stem are serving as a management service for energy companies and utilities and fit more easily into traditional SaaS business models. Because AI/software still has fairly high barrier of entries, these startups aim to provide a valuable service, while allowing traditional energy companies to focus on their core competency. At the same time, the use of AI is a value-added proposition for these companies, as it promises the ability to provide cost-savings and insights that are not easily identifiable by human expertise or traditional control algorithms.
AI for Design
AI is proving capable not only of controlling systems, but also designing complex systems to meet certain specifications. We find applications of this in a variety of areas: nTopology is a startup that we covered in ML4Sci #1 which uses AI to optimize the morphology of heat radiators, Zymergen uses AI+commercial biotech wetlabs to synthesize new molecules at industrial scales, etc. While AI is accelerating 3D printer adoption on the manufacturing side, it is also serving to accelerate the design of such products.
AI-powered solutions can also serve as a tack-on solution to traditional R&D. For instance, citrine provides AI-driven solutions to materials processing problems. In this case, they provide a software suite to help other companies R&D incorporate AI into their discovery process. Rather than having to build up in-house software expertise, citrine can provide a platform to materials-based companies.
As AI is helping enable Manufacturing-as-a-Service, it is also accelerating Design-as-a-Service. For instance, the design space of products drastically opens up as 3D manufacturing abilities improve. AI is able to search this large design space more efficiently and dream up designs that humans might not have conventionally looked for. In other cases, like with citrine, startups are aiming to bet that in a tight labor market for AI developers and software engineers, they can provide an AI-based science-specific addition to traditional R&D, as larger, older companies are culturally and structurally limited from adding in their own AI capabilities.
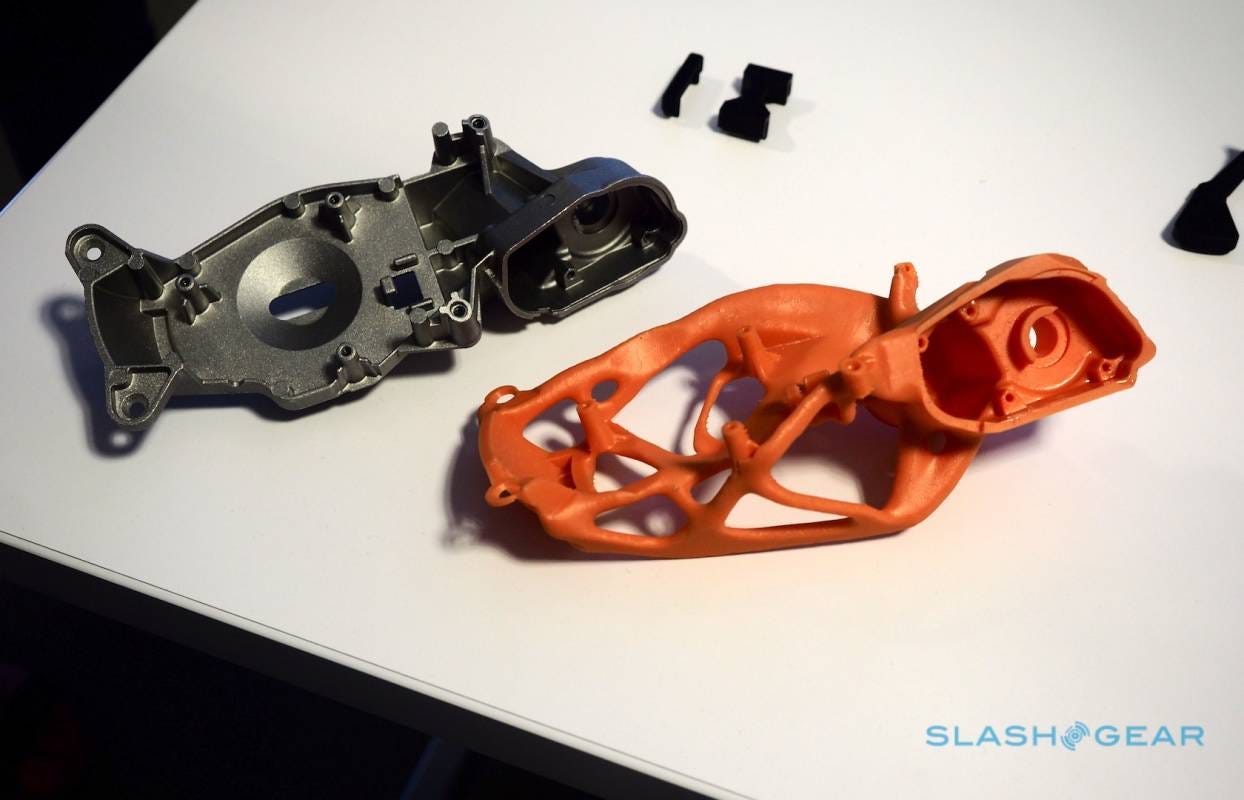
Volkswagen experiments with using AI to design lighter, cheaper, 3D printable parts
AI for Forecasting
Finally, AI is proving capable of making forecasts about the future. BlueDot, an AI startup we covered in ML4Sci #5, warned its customers about the COVID-19 outbreak weeks before the CDC did. In their case, they provide an early-warning service to corporations that want to be ahead of the curve about infectious outbreak events. As we’ve also covered, Google has begun aggressively moving into the space of weather forecasting, with models that are beginning to outperform those at NOAA. In a future where most of our energy relies on the weather, I can imagine Google offering a SaaS cloud service for accurate, real-time weather forecasts to solar and wind providers. Both of these also fit into SaaS models powered by AI.
Drop a 💬 if you have other examples of industries undergoing a similar transformation to AI-powered SaaS!
Conclusion
As science becomes increasingly driven by in silico experiments, we will see the same business trends that drove the software industries appear in science and engineering companies, namely a shift towards service-based industries. History doesn’t repeat itself, but it sure does rhyme. And the tune of the science and engineering fields is starting to sound a whole lot like that of Google and Microsoft back in the 2000’s.
In the News
David Silver, at Google’s DeepMind, was awarded the 2019 ACM computing prize. In slightly older news, Yoshua Bengio (Univ. of Montreal), Yann Lecunn (NYU, Facebook), and Geoffrey Hinton (Univ. of Toronto, Google) were jointly awarded the ACM Turing Prize, which is considered the “Nobel Prize of CS”. Notice a pattern in their affiliations? 🤔
Graphcore, one of the largest UK ML hardware startups, outlines their research directions for 2020. Good mix between hardware level and architectural level advances in deep learning.
NYT: an oceanographer asks Google AI to help with identifying humpback whale singing. A great example of public-private ML4Sci collaboration
A blog post from The Gradient on achieving the “ImageNet” moment in speech-to-text problems. Some interesting ideas on how different fields achieve “ImageNet” moments (with applications to ML4Sci as well)
Google publishes paper on using reinforcement learning to optimize chip design [h/t Import AI #191]
Thank You for Reading!
I hope you’re as excited as I am about the future of machine learning for solving exciting problems in science. You can find the archive of all past issues here and click here to subscribe to the newsletter.
Have any questions, feedback, or suggestions for articles? Contact me at ml4science@gmail.com or on Twitter @charlesxjyang