ML4Sci #21: NeurIPS Workshops; Google Flooding and Weather Forecasting; AI predicts locust breeding grounds
The coldest August in the next 100 years
Hi, I’m Charles Yang and I’m sharing (roughly) weekly issues about applications of artificial intelligence and machine learning to problems of interest for scientists and engineers.
If you enjoy reading ML4Sci, send us a ❤️. Or forward it to someone who you think might enjoy it!
As COVID-19 continues to spread, let’s all do our part to help protect those who are most vulnerable to this epidemic. Wash your hands frequently (maybe after reading this?), wear a mask, check in on someone (potentially virtually), and continue to practice social distancing.
Greetings from 2049; we just experienced one of the coldest August’s in the next 100 years:
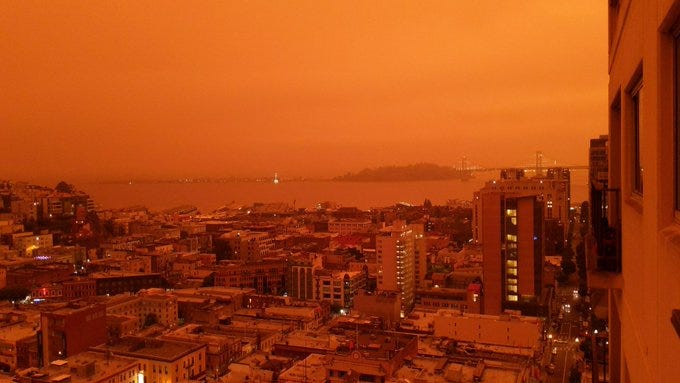
Unfortunately, I did not have time to cover in-depth several articles this week, what with the apocalypse going on and everything. Next issue, I hope to be sharing about some of the things I’ve been working on the past year or so that are all coming to fruition in the same 2 week interval (sneak peek: random forests, explainability, podcasts, and more). Enjoy an expanded In The News section this week, with some more commentary by yours truly.
📰In the News
ML
NeurIPS 2020 is approaching and this year, there are a veritable host of ML4Sci workshops: ML4PhysicalSciences, ClimateChangeAI, ML4Molecules, ML4 Engineering Modeling, Simulation, and Design (definitely the least pithy one!)
Blog-of-the-week: How to think about testing software when your software is a model
Ask HN: How does your data science or machine learning team handle DevOps?
Learning@Home - the deep learning equivalent of Folding@Home [arxiv] Decentralized compute to train massive models (and compete with large private companies that have massive compute servers)
🌊Google AI does flood forecasting for India and Bangladesh. A while back, I covered Google’s foray into AI-powered weather modelling - this most recent work supports my hypothesis that Google is using their AI dominance to move into Science-as-a-Service e.g. weather forecasting for wind/solar/agriculture, extreme weather prediction for governements, etc.
From DeepMind researchers: a collection of free, online resources to learn AI at home
❤️Detecting Deepfakes by looking at their heartbeat
Transformers are just Graph Neural Networks[TheGradient]
Science
🛰️"How satellite ‘megaconstellations’ will photobomb astronomy images”[NatureNews]
Physics Successfully Implements Lagrange Multiplier Optimizatiom[Arxiv] - a survey of all the different ways physics-based computation can solve optimization problems (and no, its not just quantum computing)
🦗[Scientific Reports] Using machine learning to predict locust breeding grounds. Another example of how AI will reshape the way we understand and interact with nature
🧬Facebook AI research updates and open-sources their 2019 paper on applying transformer models to 250M protein sequences[code][paper][tweet]
Google AI Quantum team demonstrates accurate quantum chemistry calculation on their in-house quantum computer. With quantum computing+cloud, the promise of Science-as-a-Service draws ever closer.
See more:
Paper in Science (Published August 28, 2020)
Youtube talk by one of the authors at the Centre for Quantum Technology
“Evidence for supercritical behaviour of high-pressure liquid hydrogen”[Nature][Arxiv]
From the abstract (emphasis my own):
Experiments at such extreme conditions are challenging and often lead to hard-to-interpret and controversial observations, whereas theoretical investigations are constrained by the huge computational cost of sufficiently accurate quantum mechanical calculations. Here we present a theoretical study of the phase diagram of dense hydrogen that uses machine learning to ‘learn’ potential-energy surfaces and interatomic forces from reference calculations and then predict them at low computational cost, overcoming length- and timescale limitations. We reproduce both the re-entrant melting behaviour and the polymorphism of the solid phase. Simulations using our machine-learning-based potentials provide evidence for a continuous molecular-to-atomic transition in the liquid, with no first-order transition observed above the melting line.
Myst AI closes $6M series A funding round - from the article (emphasis my own):
“The San Francisco-based startup is using AI to create a data analysis platform intended for electricity demand and supply forecasting. The new funding will be used to expand its Forecasting-as-a-Service offering.”
💊Drug Repurposing: How Often Does It Work? Historically drug repurposing has not worked - will AI remedy this or simply fall into the same problems? Important to think about given that most drug discovery AI is really just drug repurposing
The Science of Science
🎓MIT identifies 6 strategic area’s to connect the new Schwarzman College of Computing with existing departments/colleges at MIT. AI and CS are fundamentally reshaping pedagogy and interdisciplinary work - the university structure needs to adapt as well
The Future of American Industry Depends on Open Source Tech[Wired Opinion]
If you take 20-year old SAT solvers run on brand-new hardware and 20-year old hardware run on brand new SAT solvers, who wins? A paper arguing algorithmic progress provides just as much of a performance boost as hardware progress - pretty amazing considering Moore’s Law
“This scientist read a paper every day for 899 days.”[NatureIndex] Definitely resonate with the piece about the benefits of reading broadly and widely (though I am certainly not at the rate of a paper a day!)
Communicating with Interactive Articles[DistillPub] Why do we still share information as static pdfs?
🌎Out in the World of Tech
🍎Apple may be launching their own search engine
🚗 A whitehat hacker finds a series of critical bugs in Tesla’s over-the-air software service that would allow control over any Tesla model. A glimpse into the dangers of software-as-a-service colliding with the physical world and what the future of distributed cybersecurity might look
🛣️DeepMind partners with Google Maps to improve ETA prediction with graph neural networks. In other words, how data moats + rapid AI productionization can improve products and also serve as a high barrier to entry for competition.
“Taming the Tail: Adventures in Improving AI Economics”[A16Z] Why long tails poses a challenge to the economics of AI services
TikTok reveals some more details about the inner workings of its algorithm[Axios]
Policy and Regulation
🗽AI, Fairness, and Gerrymandering [ScienceMag]
Portland passes most restrictive ban on facial recognition of any US municipality
Thanks for Reading!
I hope you’re as excited as I am about the future of machine learning for solving exciting problems in science. You can find the archive of all past issues here and click here to subscribe to the newsletter.
Have any questions, feedback, or suggestions for articles? Contact me at ml4science@gmail.com or on Twitter @charlesxjyang